The Automation of Sports Writing
"Connecting those specific error patterns to long-term reader behaviors is… harder."
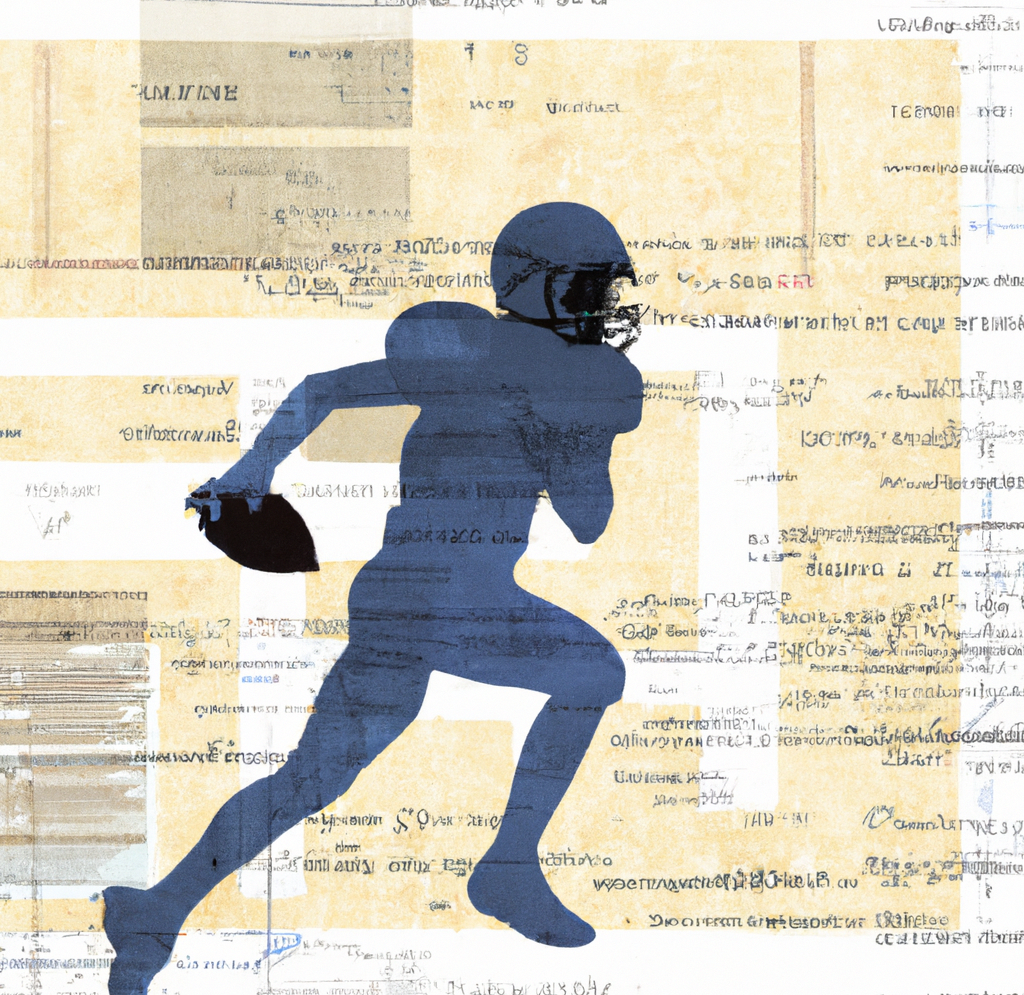
We could hardly write a blog about AI in media without at least mentioning Gannett’s Lede AI experiment, right? (I find it important to level with our audience and out ourselves as super-nerds with these tactful disclaimers.)
This all went down a few months ago, and WNYC published an excellent interview with Lede AI’s founder where you can learn about the whole thing.
Long story short:
Gannett partners with a programmatic news service for local sports coverage, people start to notice strange repeating phrases like “close encounters of the athletic kind,” templated placeholders bleeding into copy, and all sorts of the techno-tomfoolery that makes us laugh and cry. It ends with Gannett canceling the deal, people learning more about the founder’s quite reasonable goals for the service and its ambitions to offer reporters more time to conduct interviews, and less time re-writing the same clunky postgame article format a million times.
[Of note: The AP also hosts a prototype AI tool meant to automatically create the first draft of a story within a CMS.]
So why am I coming back to Lede AI now on MoP? I’ve got an ax to grind! I’ve found myself terribly annoyed with the implementation of ML in sports writing for years now.
And, look, I get it — people just aren’t reading sports in full sentences and paragraphs like they used to, not even me. I can remember a time years ago when I was actually disappointed Bill Simmons was putting out yet another podcast instead of a 12,000 word weekly epic. Now I just think: When am I ever going to make time to read a long sports thing anyways? Okay, I sometimes do. But, again, super-nerd.
And, look, I get it (part 2) — Back in my days helping run a 5x per week student newspaper, “previews” for sports stories were probably the most formulaic pieces we produced. One of my many fond Badger Herald memories is sports editors reading off their section’s agenda for the day: “Basketball preeeeview…. Football preeeeview… Volleyball preeeview… Mason coooolumn… one more… big meet this weekend… Track preeeeview”
So it makes sense that even ESPN has outsourced many of its game previews to a company called Data Skrive. Their service probably does very many incredible things for ESPN’s content products, but writing game previews is not among them. They seem to discover new, innovative ways to put statistics and performance facts in exactly the wrong context – as if specially engineered to trigger the obsessive sports fan. The result of all this? Since I can’t be sure I can trust that the content is meaningful, I don’t read ESPN game articles at all anymore!
I could gripe about sports journalism all day, but I actually do have a point here.
Let’s get back to Lede AI: What they’re doing does not exactly leverage that peak LLM magic. Sadly, not everything is Altman’s fault. This is a simpler setup. Per WNYC’s reporting, these products work by plugging given variables into pre-written templates – more or less programmatic madlibs.
While you could certainly measure an error rate by creating a test rubric and using it to score representative subsets of articles over many time intervals, connecting those specific error patterns to long-term reader behaviors is… harder. (to say the least)
On top of that, it would take a deeply artful analysis of test data to measure a critical error rate — that "stop the presses" moment, which might become terminal for an important line of business. Which, of course, is exactly what happened here with Gannett.
Per Jay Allred, who runs Lede AI, the idea is to use the author “Autonewsdesk” to do the formulaic boring high school sports preview template writing, and focus dwindling reporter resources on getting good quotes from coaches, players, etc. to put meat on the programmatic bones.
I don’t mean to pick on them at all here: A small company had a big launch with a huge company and it went bad, and they have no intention of killing entry level journalism jobs, and tech integration is a huge opportunity to build the local news business.
The interview with Allred in WNYC is a fascinating little look at this field of machine-assisted journalistic writing, and a couple of things he said really stick with me:
- Most (but not all) readers understand that what Lede AI provides its customers is simply information.
- AI cannot do journalism.
Talking about this at the coffee shop right now, Erica makes a great point: If this is not journalism, and we all agree that it is not journalism, why are we offering this stuff in a format that looks exactly like journalism?
Just about everyone on the “Paper” side of the equation here knows about Marshall McLuhan's timeless media truism: The medium is the message. The outputs of these AI journalism content systems should look like information! Charts and graphs, mapping progress for a team over time… Anything, anything at all that does not look like an inverted pyramid news article would be better than disappointing the reasonable expectation that the thing that looks just like journalism will be journalism.
Another idea to solve this: Follow a recommendation from NPR’s Scott Simon and use this budget to pay a bunch of high schoolers to write about their own damn sports.
Comments
Sign in or become a Machines on Paper member to join the conversation.
Just enter your email below to get a log in link.